Aniruddha Reddy, head of EMEA Sales, Applied Intuition, offers advice on how to best power autonomy with log data, sharing his best practices for log management ahead of his presentation on the same theme at ADAS & Autonomous Vehicle Technology Expo Europe, June 13, 14 and 15, in Stuttgart, Germany. Currently, Ani is focused on transitioning global OEMs towards virtual development and creating best practices-based methodologies. Before Applied, Ani led strategic consulting and engineering at IPG Automotive. He has a Master’s degree in the development of F1 cars from Cranfield University.
Describe your presentation?
Log data is one of the most essential building blocks of autonomous systems development. However, due to the costs and risks involved in real-world testing, ADAS and AV programs must collect and manage their log data effectively. In this presentation, we will discuss the journey of a log file, from log collection and exploration to log simulation and archival. We will also discuss the technical building blocks, ideal workflows, and cost-management strategies of an expansive log management and testing process. How have increasingly sophisticated ADAS and full self-driving systems changed the requirements for log data? As ADAS and AV programs become more advanced and the features they develop become more complex, they must significantly overhaul their platforms for log data management. For example, triaging issues from on-road testing can take significant amounts of time. The right tooling properly automates large parts of the triage process and enables the deterministic reproduction of issues in simulation. This way, teams can confirm whether the issue has been resolved without conducting additional on-road testing. Regression testing ensures that previously solved issues do not reappear. Lastly, storage costs compound as data collection increases. Teams should thus manage storage types and data archival to reduce cost.
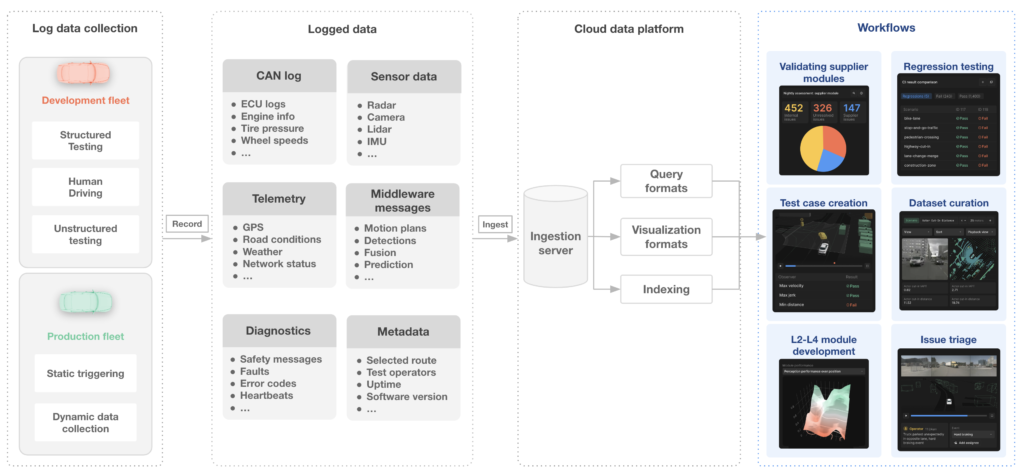
What are some common problems or challenges developers may have experienced with log data management in the past? After collecting hours of data with a test or production fleet, ADAS and AV programs often find it difficult and time-consuming to filter through the data and identify the most interesting situations. They might also find it challenging to recreate these situations deterministically in simulation and confirm whether an issue has been solved without conducting further on-road testing. With regard to cost, where would you advise developers and engineers to focus their attention with regard to log management? Costs for log management extend beyond the bill from a cloud provider. Most ADAS and AV programs incur costs from not leveraging existing data, conducting additional on-road testing, and failing to automate the triage process. By utilizing cold storage effectively as an alternative to archiving data, programs can reduce storage costs significantly. Transforming raw sensor data into a visualization format helps teams reduce storage costs, lower the latency to visualize data, and increase playback performance.
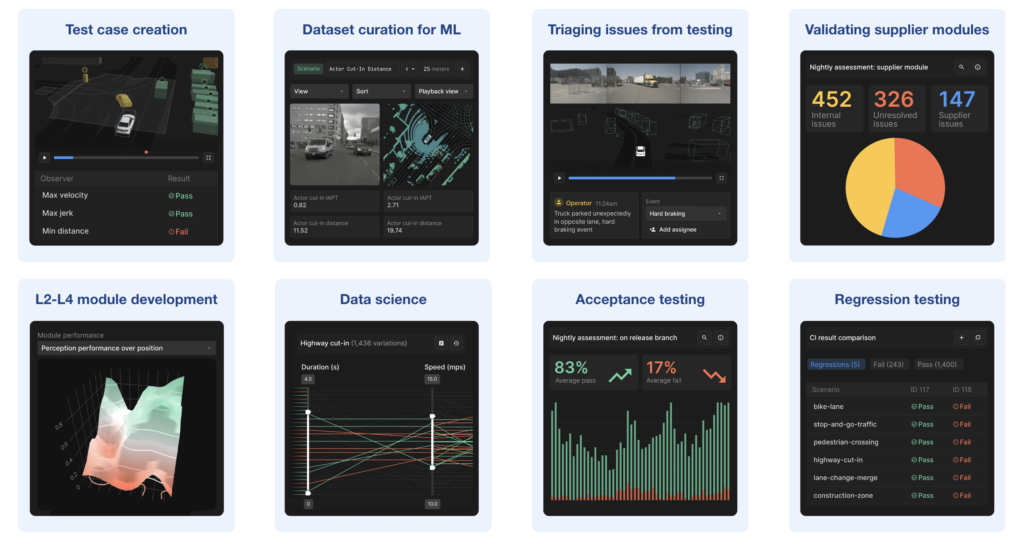
What is the key message you would like to convey at the conference in Stuttgart? Every ADAS and AV program has different needs and challenges, but log data management is an essential building block for all successful ADAS and AV development. It allows teams to leverage their collected data effectively, utilize resources efficiently, and reduce costs. Our presentation speaks to machine learning (ML) engineers, triage teams, function engineers (perception, controls, motion planning, etc), algorithm developers, systems engineers, and simulation operations teams.
Don’t miss Ani’s presentation on Day 3, Thursday, June 15, at the ADAS & Autonomous Vehicle Technology Expo Europe conference (rates apply) – see the website for the full conference program and booking form.