Raquel Urtasun, former chief scientist at Uber ATG and now founder and CEO of Waabi, explains the self-driving startup’s unique AI-first approach to delivering true autonomy, in an exclusive interview with Autonomous Vehicle International.
With US$83.5m in initial funding, Waabi made headlines across the tech world when it launched back in June, with a press release littered with the sorts of buzz words (deep learning, probabilistic inference, complex optimization, etc) that get investors and journos all in a lather. Ironically, ‘waabi’ itself translates into ‘simple’ in Japanese (as well as ‘she has vision’ in Ojibwe – a language spoken by native Americans in Ontario, home to the company’s HQ).
The Toronto-based tech firm, which also has an office in California, promises a breakthrough approach to the use of AI in self-driving, to overcome some of the inherent problems associated with current approaches to building self-driving software stacks. In some instances, companies use multiple AI systems to solve small sub-problems, while in others a ‘black box’ approach is utilized, which means the autonomy system cannot generalize to unseen scenarios. Hence the interest in Waabi, whose approach features a single AI system and is able to take the output of the sensors and produce the decision making for the self-driving vehicle.
The company is also promoting its own purpose-built closed-loop simulator for the testing at scale of safety-critical edge cases, to drastically reduce the amount of real road test miles normally required, thereby slashing costs.
Waabi’s 40-strong team will initially focus on developing software solutions for the logistics industry, with a particular emphasis on long-haul trucking – a sector in desperate need of technological innovation to deal with a chronic driver shortage and pervasive safety issues.
“Raquel is truly one of a kind – a tenacious and inspiring leader who empowers those around her to excel,” said Dara Khosrowshahi, CEO of Uber, which chose to invest in the firm, at the time of its launch. “I can’t wait to see everything she accomplishes for the self-driving industry.”
Autonomous Vehicle International was fortunate enough to catch up with Raquel – who is also a professor in the department of computer science at the University of Toronto and a co-founder of the Vector Institute for AI – to discover more about her unique perspective on the self-driving sector.
Describe your career to date, why you decided to launch Waabi, and how you have attracted so much investment.
When I studied electrical engineering in Spain, half of my class were women. That seemed very normal at the time. The reality is, men usually outnumber women in the scientific and engineering fields – women have to accomplish 10 times as much to be recognized. I’ve experienced discrimination over the course of my career, and that’s unfortunately something many female engineers still struggle with. But I never gave up. I never lost the drive to keep working hard and overcome roadblocks in my career.
Self-driving is also one of the most exciting and important technologies of our generation. As a scientist, I’m always attracted to the most difficult problems that require innovative solutions. Self-driving is a challenging field that has captivated me for years because, once solved at scale, it will change the world as we know it. I personally know too many people who have had auto accidents, and the two million deaths per year on the roads will remain high if a solution doesn’t emerge soon.
Waabi is the culmination of my 20-year career in AI and 10 years of building self-driving solutions. The more time I’ve spent in this industry, the more certain I’ve become about what it will take to actually bring this technology to life. Our AI-first approach at Waabi is unique in the industry and, I believe, key to commercializing this technology.
Our investors [which include Khosla Ventures, Uber and Aurora] are passionate about the same areas we care about. Some have deep expertise in the logistics space, which is, of course, a big focus for us; others have expertise in deep tech and AI; and others specifically support diversity in tech. The recent Series A round was a confirmation of investors’ faith in our capabilities, vision and team.
Upon launch, Waabi stated: ‘The traditional approach to engineering self–driving vehicles results in a software stack that does not take full advantage of the power of AI’. How so?
There are two main existing approaches to autonomy: the traditional, unscalable approach, which does not exploit the full power of AI but instead only uses AI to solve small subproblems within an overly complicated system; and the ‘black box’ AI-first approach, which is not explainable and/or verifiable and cannot generalize to unseen scenarios.
Neither is suitable for commercial deployment of self-driving technology.
For example, motion planning is handicapped in these types of AV approaches. Traditional motion planners rely on handcrafted features and cost functions. This results in simple decision making that can neither properly leverage uncertainty nor handle the complexity of the real world.
Testing and AV safety pose another set of problems. Structured testing at a test track facility cannot test dangerous scenarios at scale, and replaying scenarios collected from public roadways does not accurately evaluate how the self-driving vehicle will behave and react. A simulation-based testing approach does enable testing at scale of safety-critical scenarios, but current simulation systems fall very short of this promise due to their lack of fidelity, their lack of scale and their inability to test the entire software stack.
For instance, traditional simulators use heuristics and handcrafted rules to specify the scene and the behavior of traffic participants. Such approaches can be brittle and often result in unrealistic scenes since they do not accurately model the relationships between different actors, nor do they cover the diversity of the real world.
What do you mean by an ‘AI–first’ approach? How will you use it to avoid manually tuning the software stack, for example?
At Waabi, AI is at the center of the solution. What we mean by this is that a single AI system is able to take the output of the sensors and produce the decision making for the self-driving vehicle.
Our breakthrough AI-first approach, developed by a team of world-leading technologists, leverages deep learning, probabilistic inference and complex optimization to create software that is end-to-end trainable, interpretable and capable of very complex reasoning.
This, together with a revolutionary closed-loop simulator that has an unprecedented level of fidelity, enables testing at scale of common driving scenarios and safety-critical edge cases. This approach significantly reduces the need to drive testing miles in the real world and results in a safer, more affordable solution.
By ‘interpretable’, we mean that, unlike other approaches that use a ‘black-box’ AI approach, we produce interpretable representations that are explainable and allow us to trace back the reasons why the AI system made a particular decision.
By ‘end-to-end trainable’, we mean that unlike traditional approaches that require time-consuming manual tuning, the entire system can be automatically learned from data. This speeds up development dramatically.
‘Capable of very complex reasoning’ means we are able to mimic the same complex decision processes that humans carry out. This contrasts feed-forward deep neural networks that are traditionally used for autonomy, which do not have the ability to reason but only to make instantaneous decisions. In our approach, this reasoning is done through probabilistic reasoning and complex optimization.
What is your approach to simulation?
We’ve created an in-house closed-loop simulator with a high degree of fidelity that allows for testing of the entire autonomy stack. It enables us to test at scale common driving scenarios as well as safety-critical edge cases. Our unmatched level of fidelity allows us to mathematically correlate how the entire system behaves in simulation with what would have happened in the real world. This is a game-changer, as we significantly reduce the need to drive in the real world in order to understand how our system performs. Furthermore, our autonomy system can learn to act in situations we might have never seen before by training our AI-first approach in simulation.
A traffic scenario is defined by the background (buildings, trees, road, etc) as well as the traffic participants (vehicles, pedestrians, etc) and their behaviors. To evaluate self-driving vehicle safety, these scenarios must depict a wide range of traffic configurations (rush hour, for example), and replicate different traffic behaviors (tailgating, cut-ins), including those that might happen rarely. Importantly, our worlds
are not created by artists but are instead automatically extracted from sensor data, resulting in a much more realistic and scalable solution. These worlds can then be composed to create new scenarios that were never observed in the real world.
Why long–haul trucking as an initial focus, and are there any formal partnerships at this stage?
The narrative around autonomous driving in trucking has matured over the last few years. Today, it’s widely accepted that AD will, in fact, be good for long-haul trucking and truck drivers. This is an industry plagued by chronic driver shortages and pervasive safety issues – it’s actually among the most dangerous professions in the US. Rapid deployment of autonomous trucks can help solve the shortage of truck drivers and improve the quality of life for drivers by tackling the longest, most grueling hauls.
While driving on highways is certainly challenging to solve, it is still easier than city transportation. For these reasons, Waabi is focused on trucking first. Self-driving stands to make the biggest and most immediate impact in this industry, unlocking productivity and, in turn, strengthening the economy.
To answer your question about formal partnerships, I can say that yes, we will partner with industry leaders to bring a product to market. Our focus is on solving the things that still need to be solved, so formal partnerships will inevitably be part of our broader approach.
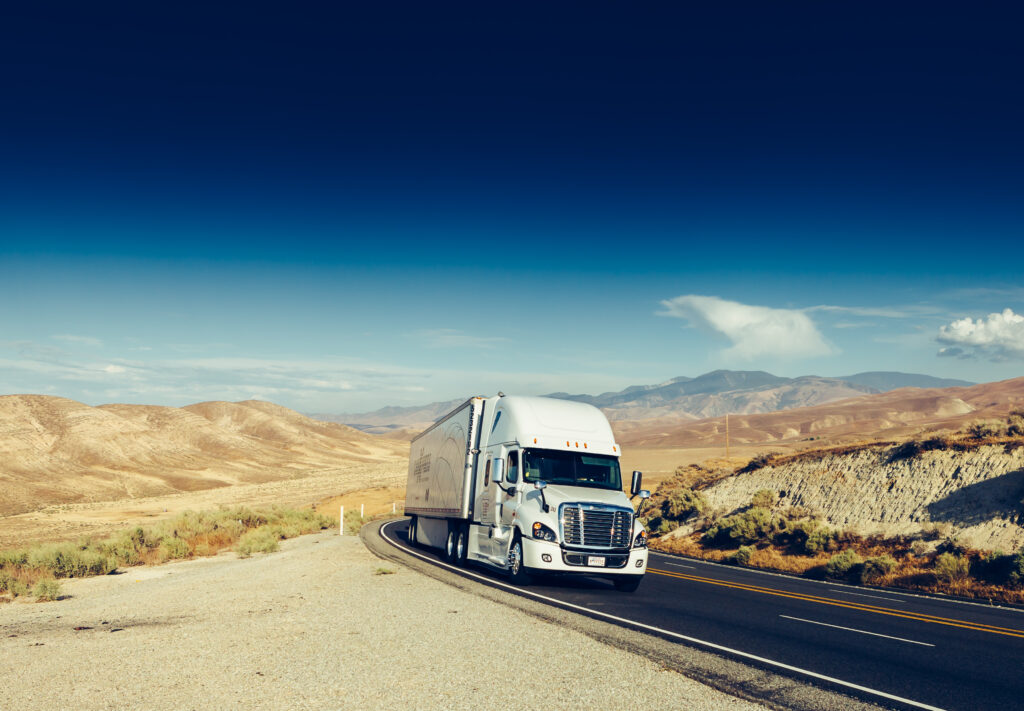
Is Aurora one such partner?
Aurora was a minor investor in our first round and has no special rights (i.e. they don’t have any right of first refusal). Chris [Urmson] and his team have been completely supportive of Waabi’s vision. We’re all working toward the same ultimate goal and we all believe passionately in the potential of self-driving technology.
Where do you look for new staff, and do you have a message for vendors?
The heart of Waabi is a team of AI experts who are considered to be at the forefront of innovation in self-driving, globally. We don’t follow in the footsteps of what others are doing or have already done. We create change with our own entirely new, unique approach.
My team is more like my family at this point. We are complementing our existing team by hiring the best talent. There is a lot of excitement in what we’ve achieved to date, and I’m so excited by what’s to come.
We have a very open approach to partnerships and want to leverage the amazing technology that has already been built, for example in sensors, computing and OEM platforms. It’s great to see the accelerating maturity of the ecosystem, and we believe we can help the commercialization efforts of many of the vendors by providing state-of-the-art autonomy and simulation environments.
This article was first published in the November 2021 print edition of Autonomous Vehicle International, which is available to read, online, here. To subscribe to receive future issues of the magazine, click here.