Tom Dahlström, business development manager, atlatec, ponders if self-driving cars need HD maps, and if so, how best to keep per-vehicle costs to a minimum.
When conversation turns to autonomous driving, one of the names that always comes up is Tesla – and of course ‘Autopilot’ or ‘Full Self-Driving’ (FSD), as their models’ driver assistance features are called. There’s an ongoing debate over what actually constitutes a self-driving vehicle, even within Tesla itself: what Elon Musk calls self-driving, his own lawyers call a mere assistance function – clarifying in communications with the DMV that “currently neither Autopilot nor FSD Capability is an autonomous system” and that there is no roadmap for them to become one.
Meanwhile, Tesla’s head of AI, Andrej Karpathy, recently gave some insights into the reasoning for their decision to drop radar sensors from future Tesla models, instead relying only on cameras for environmental perception. There’s two interesting takeaways from his statements:
Tesla claims the simultaneous use of cameras and radar produces too many perceptional conflicts, making sensor fusion and decision making problematic.
Tesla will continue to not use HD maps, because maintaining them would be a tremendous effort.
Tesla is definitely right about these problems being hard. However, the rest of the automotive industry seems to disagree with them about what the implications should be. Let’s take a quick look at the first point:
Are cameras superior to radar?
Karpathy made sure to underline that Tesla’s camera systems are the superior sensor in basically all situations – without releasing any data for independent review – which would make radar a source of unnecessary error and latency. This is something that many other car makers disagree with: radars are a rather ‘physical’ sensor, meaning their measurements (detected reflectivity and time of flight) can quickly be interpreted as objects and their distance without having to run the input through lots of algorithms. With camera data it’s the opposite: the recorded pixels themselves contain no data about what is where in a picture before undergoing computer vision processing and algorithmical segmentation.
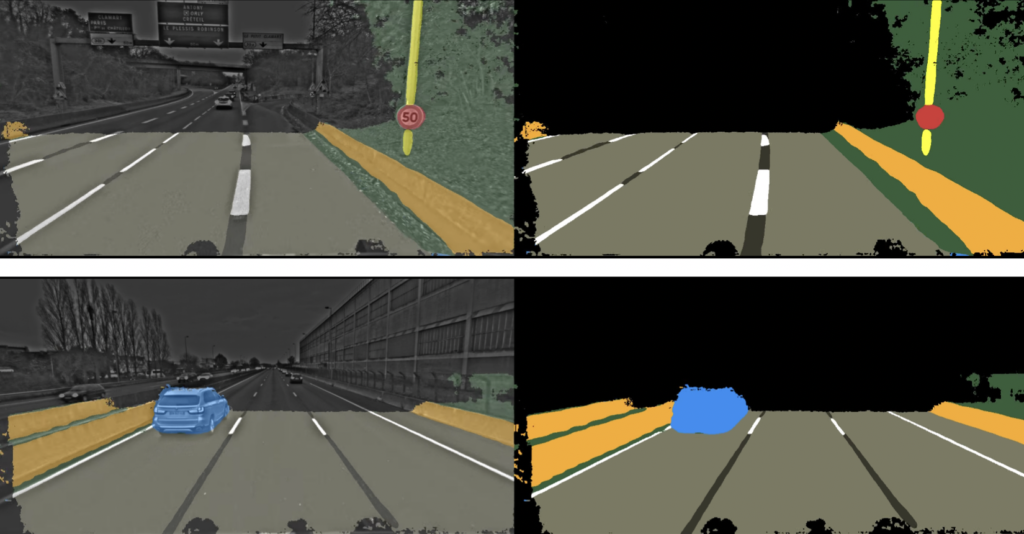
Additionally, both systems are good and bad at different things and in different situations, making their combination a good compensation for each other’s weak points. Since this is pretty much consensus among every OEM other than Tesla, and radar has been mainstream in series production vehicles’ assistance systems for quite some time, let’s table this for now and look at the other issue in play: HD maps and how to maintain them.
Do self-driving cars need HD maps?
In his presentation, Karpathy correctly calls HD maps a part of the infrastructure for self-driving cars: much like engines require infrastructure in the form of gas or charging stations to operate at scale, self-driving features require HD maps to precisely localize themselves and to accurately navigate on a lane-level basis.
In case you are not familiar, HD maps (high definition maps, also called 3D maps) are roadmaps with inch-perfect accuracy and a high environmental fidelity – they contain information about the exact positions of pedestrian crossings, traffic lights/signs, barriers and more. This is necessary for autonomous vehicles because they cannot compensate for map inaccuracies the way humans do when following their GPS: If a map is a meter or two off, a human driver is not going to crash because of it – we simply understand what the map refers to in the scene we see through the windshield.
An autonomous vehicle, however, cannot deal with such inconsistencies and the high complexity of environment and traffic that easily. If you’ve ever gotten mad at your laptop, thinking “Why doesn’t it simply do what I want it to?!”, then you know how hard it can be for computers to understand things that humans consider basic or self-explanatory.
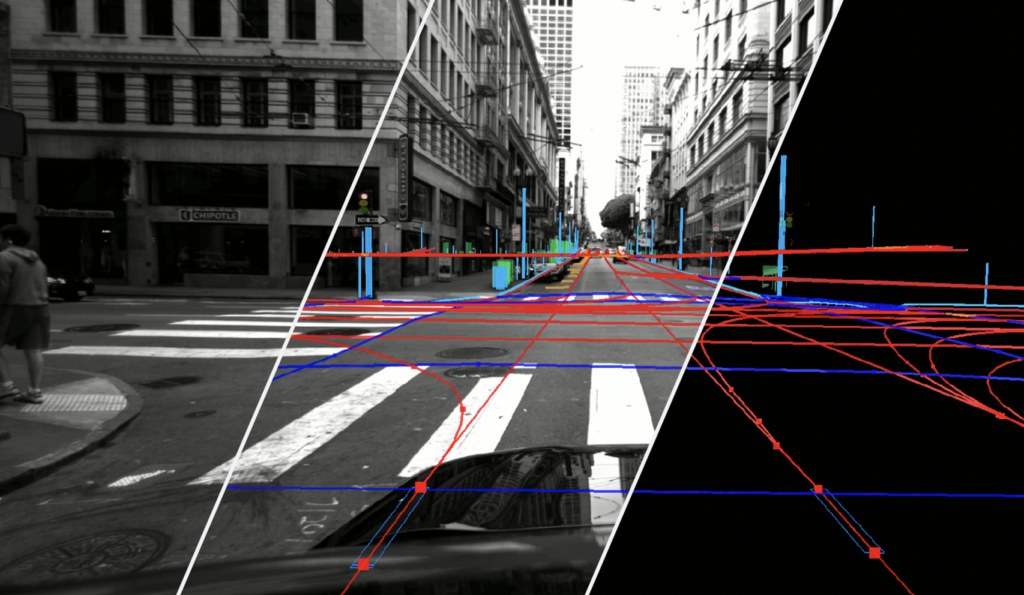
A few years ago, it was still an open question whether HD maps were going to be part of the solution for this problem: Karpathy is correct to say that making and maintaining these maps is hard, especially at the required scale. However, over the last couple of years every other car maker has come to the realization that any system above SAE Level 2 will require the use of onboard HD maps – for safety reasons as well as for driver/passenger comfort. Real-time computing and onboard sensors alone are simply not powerful enough to master the complexity of our roads and the traffic on them.
It’s helpful to think about HD maps as the difference between knowing a route by heart and driving it for the very first time – and thus between getting into a car with a seasoned chauffeur or a driver completely new to the city. Karpathy actually says as much himself about Tesla not using HD maps: “Everything that happens, happens for the first time, in the car, based on the videos from the eight cameras that surround the car”.
A very hard challenge indeed: a look at some of the first videos showing the performance of Tesla’s newest release of Full Self-Driving, FSD 9, shows the vehicle making multiple mistakes, including driving across bus lanes and crossing solid lines into the wrong lane.
If an OEM like Tesla releases a system prone to such errors that would be mitigated by HD map data and doubles down on not using HD maps in the future, it seems fair to think that they’re indeed a very challenging concept. At the least, it warrants a closer look at the details involved.
What makes using HD maps a challenge?
So what is it that makes using HD maps in series production vehicles so hard? After all, there’s companies out there that have been producing them for years – including big names like Here Technologies, TomTom and of course Ushr, which provided 130,000 miles of HD maps of US highways for General Motors’ Super Cruise system several years ago.
There’s multiple reasons HD maps haven’t become a no-brainer yet – chief among them are the costs and time required to create them, and to keep them up to date.
Mapping out a continent’s entire highway network (which is the scale required for mainstream vehicles) is a seven-figure investment, at least. Depending on an OEM’s predicted fleet size and take rates for the feature that utilizes the maps, the resulting per-vehicle costs are not negligible – especially in an industry like automotive, where traditionally every little bit of margin counts.
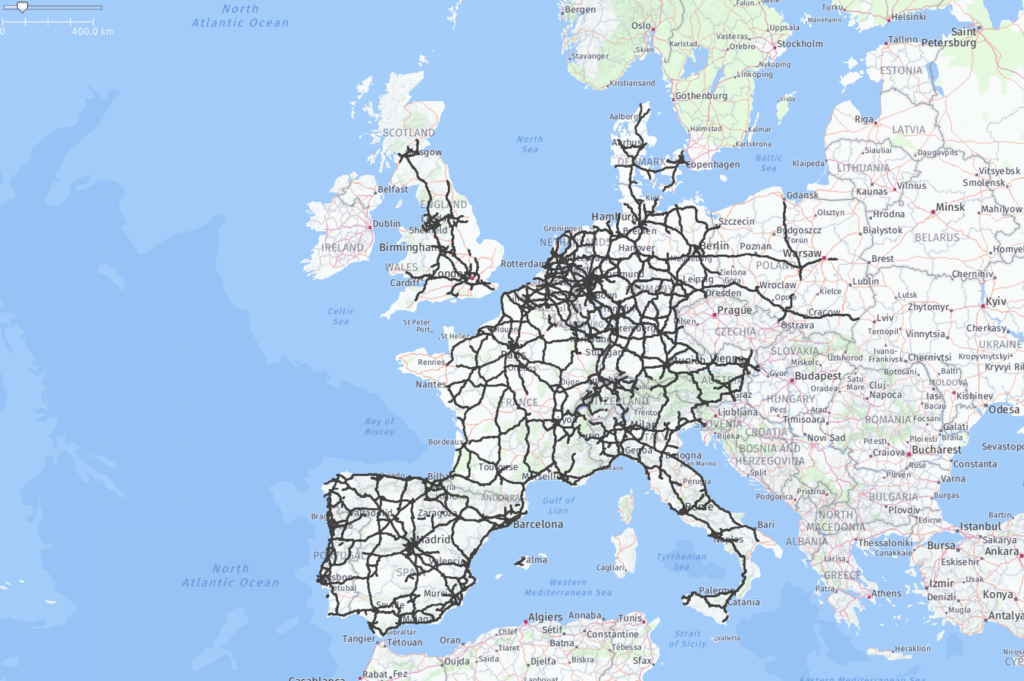
And once that initial batch of maps is done with production, parts of it will already be outdated due to roadworks and similar. If your Level 2/Level 3 assistance system depends on up-to-date maps to be engaged, this means you need a pretty short turnaround time for updates – a few weeks at most, down from the multiple months we’re familiar with for SD maps (standard definition) that are used for human navigation.
As a result, you need a solution to not only map out 100,000+ miles of road, you need one that continues to efficiently re-map it wherever and whenever necessary – without compromising on quality and without breaking the bank.
For most of the companies that have been working on HD maps for a while, these requirements are a bit at odds with the usual approach: the norm is to use purpose-built survey vans or SUVs, loaded with high-end sensor hardware and running above US$200,000 or even US$300,000 apiece. These vehicles need to be utilized heavily to generate an ROI for their owners; and they make it economically impractical to operate a fleet of the size that would be required to quickly re-scan small parts across the USA or Europe for updates.
So in a way, Tesla’s analysis is correct – if you’re only looking at the solutions traditionally used for HD maps production. However, new technology to build and maintain them at the required scale and within the expected time and cost limitations already exists today.
The wisdom of the many: crowdsourcing HD maps
In 2017, chip maker Intel bought an Israeli company named Mobileye for US$15.3bn – the biggest acquisition of an Israeli tech company up to that point. Mobileye is an automotive supplier that produces (among other things) camera systems that power driver assistance functions in series production vehicles, such as collision avoidance and blind spot detection systems. Their real value, though, lies beyond the company’s hardware:
According to their own data, Mobileye systems are installed in more than 300 vehicle models by 27 OEMs, which makes for a good probability that most main roadways are being driven on by at least one of their cameras somewhat regularly. Leveraging this fact, Mobileye harvests the sensor data from customers’ vehicles in the cloud, aggregating them into what is to be a crowdsourced, constantly updated map database without input from a single dedicated survey vehicle. In other words, the systems’ end users become their own data suppliers.
There is currently no mainstream Level 3 system operational that relies on Mobileye’s approach to guarantee safe hands-free operation of a vehicle – yet. The cameras (and other sensors) used in series production, and their data, deliver much lower accuracy than what an HD mapping system would use, and it’s unclear how much of the processing/annotation process can (or should) be fully automated.
The technology may still be young, but the concept of Mobileye’s approach is pretty much recognized as where things are heading: several OEMs and Tier 1 suppliers are working on their own versions of a crowdsourcing system for HD maps to expand and round out their portfolio. Toyota’s Woven Planet, for example, just announced their acquisition of mapping company Carmera, after it tech company Nvidia announced earlier this year that it was buying DeepMap, further underlining its ambitions in automotive.
So if Level 3 vehicles are hitting the market in 2021 and 2022, but crowdsourcing HD maps is still several years away from truly becoming mainstream, how will they be sourced in the meantime? That’s where another approach to HD mapping comes into play …
Bridging the gap: how to use HD maps at scale today
This is where we come back to computer vision, Tesla’s weapon of choice over other sensors. The domain of HD mapping today is indeed one where this sensor approach can really shine: Cameras are a long-established technology, and good quality hardware is available for cheap. Also, using stereo vision (two cameras looking in the same direction but mounted apart from one another) enables the computation of 3D depth images with higher resolution than many other sensors would produce.
This combination enables the replacement of highly expensive hardware and special-purpose vehicles with low-cost equipment – and high-performance software processing. Where Tesla has to compute “everything that happens […] for the first time, in the car”, HD mapping companies are not currently faced with such challenges.
For the foreseeable future, there is no expectation for mainstream vehicle assistance functions to be powered by real-time HD map updates. So we can still use smaller fleets, with much better cameras, GPS and motion sensors, than OEMs bring into series production – and we have the luxury of being able to process their data in post-production, with complex algorithms that require time to run.
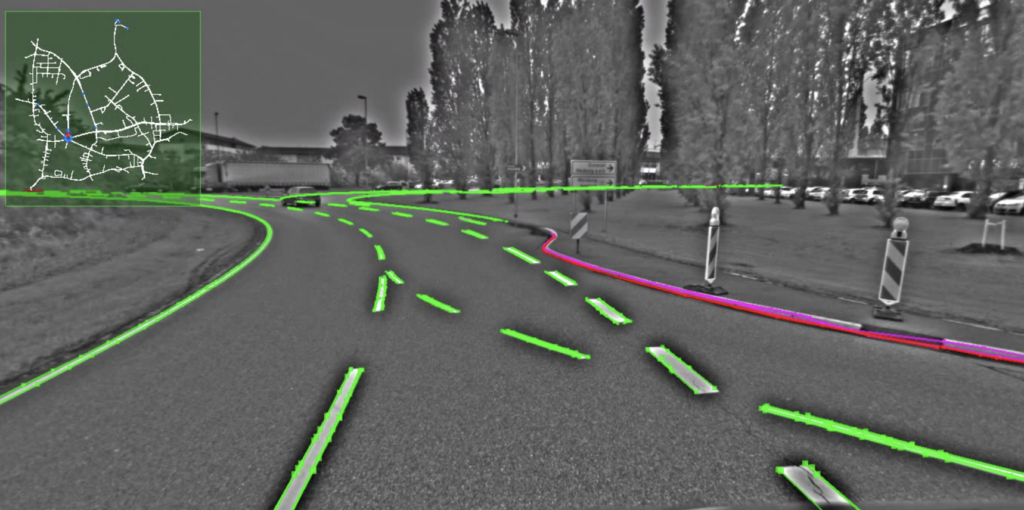
So how about the business case – is it really possible to get around the high costs for a survey fleet? The answer is yes, if you take advantage of the fact that you can strip down surveying hardware and build a system that is easy enough to use without specialized engineering know-how.
If you can make your equipment vehicle-independent and easy enough to operate for a layperson, you unlock a powerful benefit: you’re able to use part-time drivers and their private vehicles. The working model becomes the same as for the people driving for AAA or Uber; many of them have other daytime jobs and only take select calls in-between, when they’re dispatched. If anyone with a driving license qualifies as a survey driver and they have access to low-cost, self-contained hardware that can turn any car into a survey vehicle, you have a mapping fleet. This enables the delivery of the map update requirements that OEMs are already facing today, and at a cost that is compatible with automotive pricing requirements.
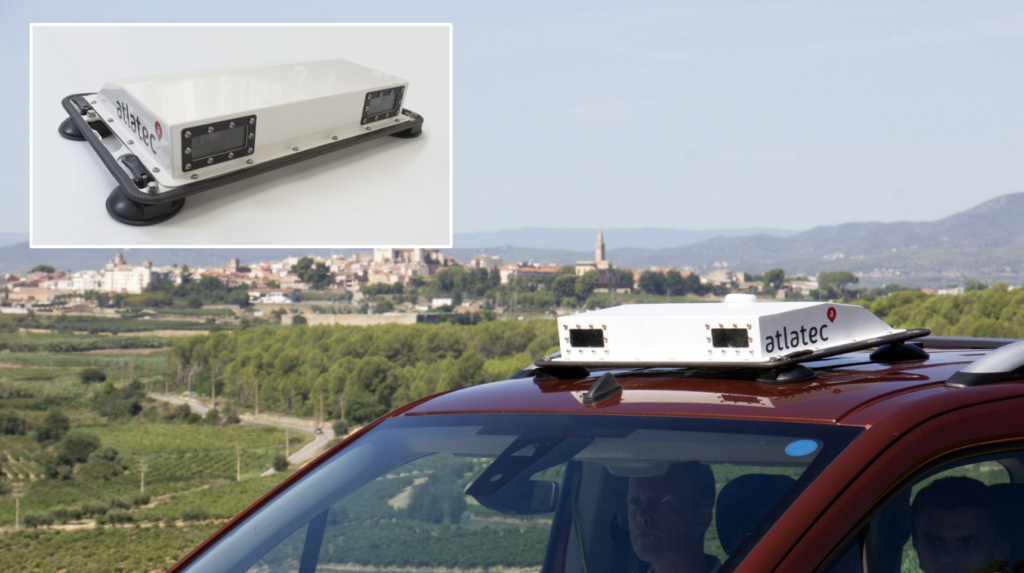
Make, maintain, mass-build: a map to series production
Based on all this, one can distinguish three likely phases for how HD maps will be produced over the coming years. Let’s call it the MMM model:
Make. Creating the first version of HD maps, at the very least for all highways across the USA, (most of) Europe and Japan. This coverage will be the minimum requirement for features that are supposed to go into series production with a relevant take rate. Most contracts for this type of work will probably be executed within the next 12-24 months.
Maintain. Keeping this first version of your HD maps up to date – very likely still using HD-grade survey equipment and processing methods, but at low cost and with shorter turnaround times (~2 weeks from the time a change becomes known). Since this approach is feasible with today’s technology, there’s no reason not to use it for several more years, buying time for the final step.
Mass-build. Designing and implementing an end-to-end solution that allows the harvesting of production vehicles’ onboard sensor data, reliably aggregate and process it in order to detect changes in the mapped environment, and update the map accordingly and stream it back to the fleet. This will likely be the ‘holy grail’ of HD mapping in this decade, and it will take quite some years to build.
Mobileye seems to be pretty far ahead of many other players today and might pull off step 3 without the stepping stones the MMM model provides. But if the past is any indicator for the future, others will catch up. Automotive is one of the most competitive industries out there and there is never only one supplier for anything for long. And for OEMs and Tier 1s looking to establish competing offers, it’s good news that there’s a solution to buy the time for doing that, without sacrificing a quick go-to-market in the meantime.
It’ll be interesting to see which approaches and philosophies future crowdsourcing players favor. Mobileye’s current strategy and the development of other market actors is reminiscent of the early competition between Apple and Microsoft. On one side, there’s a closed end-to-end system that delivers high performance but that remains a ‘black box’ of sorts for its users, not allowing customization or individualization besides what’s designed by the manufacturer.
On the other side, we are likely to see a more open ecosystem, with different types of companies collaborating: car makers, sensor makers, software specialists, mapping and telecommunications companies, and each bringing their expertise to the table.
At this moment, writing this on a Linux laptop, connected to an open internet via an Android smartphone, I am very excited to see the open mapping solutions we all will create together.
About atlatec:
atlatec is a German-born HD mapping company. Founded in 2014, it employs 40 people between its headquarters in Karlsruhe and local offices in Michigan, USA, and Tokyo, Japan. atlatec’s clients include automotive companies including Ford, Volkswagen, Rivian, Continental, Bosch, ZF and more.