Virtual testing is a linchpin in the delivery of cost-effective ADAS and AD technologies, but how can engineers integrate it effectively into the development process while ensuring robust validation, asks Alex Grant in an exclusive feature first published in the September 2024 issue of ADAS & Autonomous Vehicle International magazine.
Automation is positioned at the crossroads of competing trends within the automotive industry. Arguably one of the most significant technological challenges in the history of road transportation, it’s evolving during a period of increasingly tight budgets, short deadlines and shifting regulations. Virtual testing helps alleviate the development bottlenecks but it isn’t a catchall and there are still plenty of gray areas to overcome.
“Simulation has to play an important role to cover all possible scenarios – especially the edge cases,” comments Andreas Richter, engineering program manager for operational design domains at Volkswagen Commercial Vehicles. “Conducting thousands of test kilometers on the same roads, again and again, represents only a small set of variations. Simulation can broaden this set to boundary conditions, but real-world testing will be necessary to verify a sample.”
Richter adds that the capabilities of these tools are expanding as more storage and computing power becomes available for simulation and data analysis, but says there is still room for improvement. Simulations can struggle to realistically recreate complex road layouts, traffic behavior and environmental conditions (such as weather and materials), and there is a need for improved sensor models too.
“The automotive industry also needs to understand what kinds of boundary scenarios have to be combined to get a valid test result, or which pieces can be tested separately to reduce complexity,” he explains. “Current simulation frameworks are focusing on ADAS and already having difficulties covering all relevant topics. Autonomous driving systems (ADS) are multiple times more complex than ADAS and the general idea is to apply the same simulation toolchain. It is obvious that there are some additional gaps to bridge.”
Tool suppliers are taking those challenges seriously, as rFpro’s technical director, Matt Daley, explains. The company’s ray-tracing engine is designed to create a more realistic environment for sensors to perceive, including reflections, shadows, motion blur and rolling shutter effects from cameras, which requires a move away from real-time simulation. That environment also includes additional ground truth, such as functionally accurate, individually classified markings and signage and splines to support pathway planning.
Correlation with real-world data is key. rFpro is part of two projects funded by the UK government’s Centre for Connected and Autonomous Vehicles (CCAV): in Scotland, for a period of three months, Sim4CAMSens is gathering weather data, which will be compared with the virtual environment; and DeepSafe is studying how sensors perform in dynamic situations – near misses – with the goal of commercializing realistic training data for autonomous vehicles.
Daley explains, “We have created a role of simulation performance engineer, whose sole job is to document, characterize and compare real-world data against our simulation. We’re dedicating our own resources to producing a simulation handbook. It’s about declaring the physics of your approach and the underlying models, then proving that the simulation output matches that model, which is correlated against an academic paper or similar, outlining the right way to model fog, for instance.
“You have to have a correlated, high-fidelity simulation that is going to get you accurate results that match what the real systems would do. Unfortunately, that often doesn’t come hand in hand with very simple, easy or fast simulations to run.”
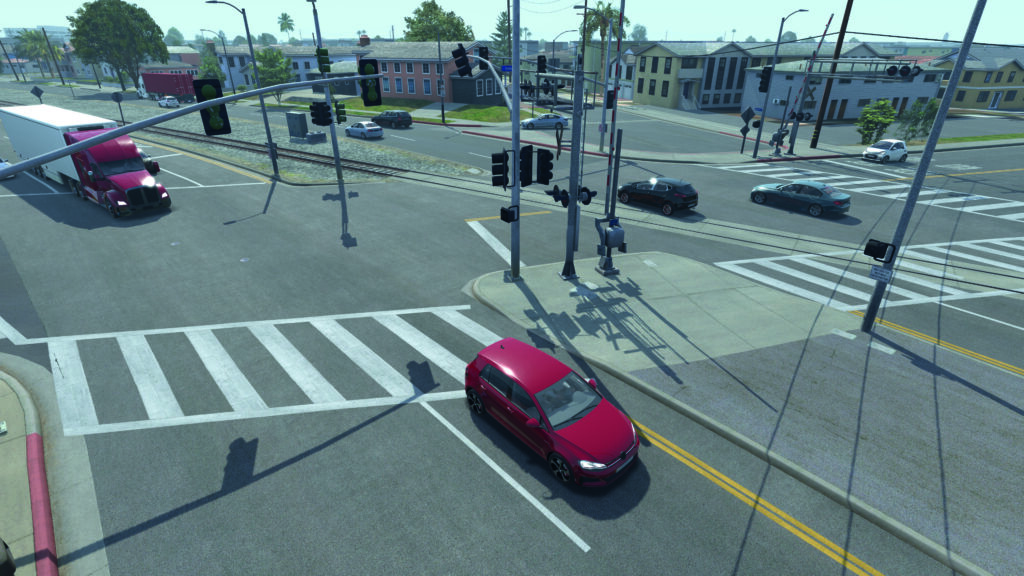
Lack of standardization
Nora Harlammert, a consultant at dSpace, agrees that conventional testing is no longer suitable for automated functions, due to the scope of the code required and the breadth of scenarios that the systems will face. Although virtual testing can help to extend that scope and discover problems earlier in development – a fact that the regulations recognize – that process relies on a continuous feed of real-world data and parallel testing to correlate the results and ensure the operational design domain (ODD) has not changed.
“An additional V-cycle for model validation must be run through to ensure traceability throughout the entire product lifecycle,” Harlammert comments. “For example, requirements for the simulation environment must be defined in parallel with technical product requirements in an ALM (application lifecycle management) tooling. In addition, data must be continuously collected in test drives to ensure efficient verification and validation of the system under test (SUT) as well as the models and simulations.
“Nevertheless, most elements are already available, but transparency needs to be clearer through process harmonization, which also supports the creation of homologation documents.”
Gil Amid, co-founder, chief regulatory affairs officer and VP of operations at Foretellix, explains that, unfortunately, there are limited standards and guidelines in place. Effective testing relies on a carefully defined ODD – a system specification, including the conditions in which the system can operate, against which its behavior can be assessed. Today, there is no standardized way to do this.
“There is one attempt at standardization by ISO – ISO 34503,” he says. “This is not fully detailed and it is often expected that the user will extend it with additional attributes and characteristics. There is work on a similar standard in SAE – J3259 – but, other than that, there is no formal industry agreement about the set of characteristics to be specified.”
Engineers also face challenges integrating that ODD into their testing and validation process, adds Amid, highlighting the importance of the OpenODD project at ASAM (Association for Standardization of Automation and Measuring Systems). The goal is a standardized, exchangeable format enabling system behaviors to be tested and measured against that specification. This is expected to deliver an OpenScenario DSL – a domain-specific programming language for merging ODDs and scenarios.
Gray matters
Marc Pajon, consultant and president at Taktech, points out other question marks in the development process. There are numerous initiatives to create standardized scenario libraries in Europe but, to date, sharing between industrial and academic players remains complicated because each has defined its own way of classifying and exploiting its database. OEMs work with scenario databases such as ASAM OpenScenario, as well as with their own alternatives. European standardization is a long way off, he says, highlighting two key gray areas.
Pajon was previously head of testing and measurement technologies for the Renault Group, where he set up the ADAS validation system and developed the ADScene SaaS scenario platform for validation and homologation of ADAS AD, in partnership with Stellantis. “Some see artificial intelligence as a way of speeding up the market launch of highly automated vehicles,” he says. “However, there are still major issues to be resolved in this area. If an accident occurs, we need to be able to explain the causes. With AI, we can develop a process that leads the driving system to make decisions, but when the accident has already happened, it’s impossible at this stage to understand the mechanism that led to the decision. There are national and European research programs that will doubtless one day enable us to trust AI, but here again, we’re in for the long haul – and that’s a real difficulty.
“The second issue is the development of algorithms. The aeronautics industry is already using self-certifying algorithms right from the design stage to develop certain safety-critical software, such as flight controls. These advances could rapidly benefit the automotive industry. Indeed, control software for an autonomous car and that for an aircraft lead to comparable criticality.”
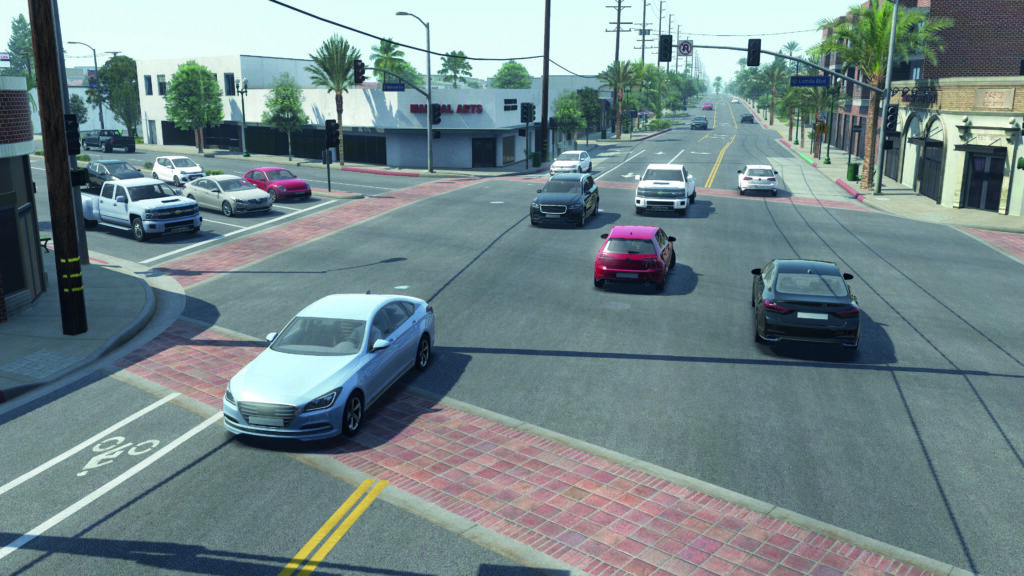
Daley points out that suppliers are already required to prove that their solutions are suitable for customers’ use cases. EU type approval regulations include principles for ‘credibility assessment’, requiring suppliers to demonstrate that their modeling and simulation are capable, accurate, correct and suitable, while also setting out the training and experience needed to use their systems. However, he adds, there are no specific criteria for tools – a detailed handbook and customer collaboration will be important to ensure tools are only used where they are designed to be used.
“The user has to define where simulation needs to be used by defining the ODD,” Daley explains. “We as a tool provider need to cross-reference that and be explicit, saying there are areas of your ODD where this simulation is not valid. We must be able, as responsible suppliers, to understand the limits of our own simulation and clearly communicate that to users.
“You don’t overstep and go into that region where you still need to do some physical testing to prove out your system. If you do that correctly and responsibly, as both developer and supplier, consumers will be much more confident that what you’ve done is valid. You create user confidence by that openness, knowing the limitations of each type of testing and declaring them when you present your safety case.”
That approach differs in the US adds Amid, where manufacturers self-certify instead of facing type approval requirements as they would in the EU. It’s a strategy that provides more scope to take risks and advance the technology, but requires a different approach to trust. With strict NHTSA requirements to openly report incidents, and the threat of expensive litigation if those processes are not robust enough and lead to a crash, US developers tend to issue public reports about their simulation capabilities to ensure transparency.
“Manufacturers don’t have full trust [in simulation], and they should not have,” Amid comments. “You would never release such a system based only on virtual testing, and I think that’s the right approach. Regulators understand that they will need to trust virtual testing but they don’t understand how. This is why in no regulation today will you find guidance on correlation.”
Pajon believes the optimum test/simulation relationship has not yet been achieved. He says that simulation is an essential tool for delivering autonomous vehicles at an acceptable cost for mass-market applications, which would enable this technology to make a big difference to road safety. However, he recognizes that virtual testing will complement physical methods rather than replacing them.
“Today, physical testing is the only recognized means of homologation – you always have to test at the end,” he explains. “It’s about optimizing this approach between testing and simulation, and making progress on confidence in input data to improve the impact of simulation on the whole process.
“I’m convinced that there’s an optimum to be found between virtual, hybrid and physical testing, which achieves the best time-to-market at the lowest cost. If you want to win over mainstream OEMs, we need to dramatically reduce the cost of development as urgently as the sensors themselves.”
Sensor data
Despite an increasingly sophisticated virtual environment, providing high-fidelity sensor models capable of simulating electronics and processing within the chip continues to be a challenge.
However, suppliers are working hard to close any gaps. For example, dSpace recently integrated Hesai lidar models into its Aurelion sensor simulation solution. Developers can now easily access Hesai lidar models via Aurelion, reducing the costs for training corner cases, while ensuring AVs can be developed, tested and validated more quickly.
Taktech’s Marc Pajon believes partnerships such as this will be invaluable. Sensors are supplied by Tier 1s, and virtual models are pared back to protect intellectual property, which can undermine OEMs’ confidence in the resulting simulations.
“What could be interesting is having a partnership between simulation suppliers and Tier 1s to generate surrogate models that will not exactly represent the physics but will be equivalent in terms of answers to the real sensor,” he explains. “This would be progress, but it requires a top-notch partnership to build those models, so that they could be in the catalog of the simulation supplier and used by all [OEM and ADS developer] customers.”
This feature was first published in the September 2024 issue of ADAS & Autonomous Vehicle International magazine – you can find our full magazine archive, here. All magazines are free to read. Read the article in its original format here.